The rise of AI has been swift, but must captive insurance accept the technology as an inevitability and adapt, or resist?
The big question
Let us begin with ChatGPT. It is, afterall, the most popular artificial intelligence (AI)-powered chatbot, which is operated by OpenAI and was recently the subject of an Elon Musk-orchanstrated US$97.4 billion takeover bid.
Ask ChatGPT the following question: “How can captive insurance benefit from the rise of AI?” Mere seconds later and the chatbot produces a detailed response, making a compelling case that benefits could include, among many others, improved risk modelling, predictive analytics, automation of claims handling, fraud detection, tailored insurance products, dynamic pricing models, and process automation.
Of course an AI-powered chatbot would find numerous applications and benefits for itself, would it not? But the insurance industry is similarly positive. Earnix, after surveying 431 insurance executives, revealed in late 2024 that insurers expect the impact of AI to nearly double from the last year to the current year, and then more than triple in the year ahead — a nearly six-fold increase in just a three-year window.
Earnix also found that 70 per cent of insurers expect to deploy AI models that make predictions based on real-time data in the next two years. However, rather tellingly, less than one-third (29 per cent) reported that they use AI models today, and the remaining one per cent said that they had no plans to use AI at all.
The rapid rise of AI is hard to ignore but the insurance industry appears to be adopting a wait-and-see approach, holding off from big and costly investments with the valid and useful scepticism it is renowned for. The captive insurance industry is similarly cautious.
Returning to ChatGPT’s response, the implementation of AI in claims automation and analytics could produce a compelling use case, not least because it would cut through the hype and deliver tangible improvements rather than revolutionary upgrades.
Gabriel Weiss, CEO and co-founder of XN Captive, which provides end-to-end captive insurance services, comments: “AI has the potential to significantly improve efficiency across claims handling and the broader captive insurance operation.”
Some of the areas where it could unlock value include enhancing data collection and synthesis.
Weiss says: “One of AI’s most immediate benefits is its ability to collect, summarise, and synthesise data, ensuring claims adjusters have the right information at their fingertips. By structuring and presenting relevant details in an easily digestible way, AI allows adjusters to make faster, more informed decisions.”
It could also automate straightforward claims processing, where the decision is clear-cut, dramatically reducing the need for human oversight. Weiss says: “This not only speeds up the claims process but also frees up human experts to focus on complex cases that require deeper analysis. While human oversight remains essential for quality control and regulatory compliance, the majority of routine tasks can be automated, improving overall efficiency.”
Work smarter not harder
Ultimately, the human touch is crucial to improving the AI technology implemented within a claims processing environment. Weiss says: “AI models can improve over time by learning from human experts. A well-designed system can compare AI-generated decisions with human adjustments, using those differences as a feedback loop to refine its reasoning. As AI is integrated into claims workflows, it will become increasingly sophisticated, enabling more precise and confident decision-making.
He continues: “Beyond claims processing, these principles can extend to other areas of captive operations, such as underwriting, policy management, and compliance monitoring. The key to success is designing processes that balance automation with regulatory adherence and customer service excellence.
“By embedding AI into core workflows with continuous learning mechanisms, captive insurers can achieve a more efficient, scalable, and high-performing operation.”
?Trent Harper, president of Breckpoint — an Acrisure partner and self-funded insurance provider offering programmes across employee benefits and property and casualty lines — notes that recent AI applications have markedly improved capabilities in underwriting, reserve setting, and workflow optimisation.
Breckpoint’s AI-driven process has cut manual report reconciliation time by roughly 40 per cent, resulting in faster, more accurate risk assessments.
But, he says, AI still has limitations in understanding the complexity of plan designs (co-pays, co-insurances, in and out of network, etc) and “we have yet to have a breakthrough in plan analysis with AI only — humans in the loop are still required”.
“Thus AI, though showing improvement, is not a replacement for a well-trained underwriter or claims specialist in many tasks. It's about finding those tasks that AI can do well, and maximising accordingly.”
Joel Raedeke, chief technology and data science officer at Broadspire, the third-party claims administrator subsidiary of Crawford & Company, highlights AI’s own ability to get right to the point as a potential benefit: “One interesting use case for AI language models is helping people to find something that is very difficult to locate.”
He further explains: “If a claim comes in the door with 40 pages of insurance policy documents and disclaimers that you must read through to find out whether a crack in the sink is covered, the AI can read those documents instantly and find that information for you.
“It can even proactively read the fact that the accident description says ‘sink crack’ and go look at the policy documents so that the moment you turn on your computer and look at the claim, it can tell you, ‘I read this for you and I think that this claim is covered and I'm going to point you to the very sentence that says sinks are covered under this 40 page contract.’ That's an excellent use case.”
AI could also identify claimants with certain comorbidities that are not specifically listed anywhere but can be detected within the data, providing a better claimant experience and reducing insurer cost.
Raedeke adds: “For instance, there might be a note in a medical record that a patient is having trouble getting out of bed in the morning and a second note in the same file indicating that they no longer enjoy favorite sports or shows.
“If you ask the AI whether there are any signs of depression for this claimant, it can surface these symptoms even if the word depression is never mentioned. Proactively finding key indicators like this can help ensure better management of the claim.”
The future of AI
Whether or not AI gets its figurative hands on a captive’s data, the captive must ensure seamless data ingestion to keep operations lean and resource-light.
Harper says: “It’s critical. Seamless data ingestion minimises manual processing, ensuring that our systems receive clean, timely data. This efficiency keeps operations lean, reduces overhead, and allows our teams to focus on strategic decision-making rather than manual administrative data cleaning and entry.”
Jonathan York, chief technology officer at XN Captive, agrees: “Captive management, like any knowledge-driven business, relies heavily on high-quality data to inform decision-making. The way data is handled directly impacts the accuracy, timeliness, and overall effectiveness of those decisions.
“A well-structured approach to data management ensures that companies can extract maximum value from their information. This starts with organising and managing documents efficiently — keeping track of unstructured content, making it searchable, sortable, and accessible. The next level involves leveraging tagging and metadata to describe the content, enabling faster and more effective retrieval.”
Advanced data extraction techniques, meanwhile, can transform unstructured content into structured data, allowing for deeper analytics and better reasoning. York continues: “The key question then becomes how to do this efficiently — manual processing is slow, labour-intensive, and prone to errors, whereas automation, powered by modern AI tools, streamlines the process.
“The latest generation of AI models can not only automate document management and data extraction but also perform complex semantic reasoning tasks. For example, AI can instantly compare thousands of insurance policies, identifying inconsistencies or key differences in seconds — something that would be virtually impossible for a human team to accomplish at scale.
He explains: “By applying modern technology to data ingestion and management, captive insurance firms can deliver a significantly better client experience, enhance decision-making, and reduce operational costs — all while handling data at a speed and scale that manual processes simply can’t match.”
Above and beyond doing what a captive does now, but better, AI can also deliver strategic gains thanks to the huge amounts of detailed claims data at their disposal.
Harper says: “AI can transform raw claims data into strategic insights. By uncovering hidden patterns and predictive trends, advanced AI tools can enable proactive cost containment, refined underwriting strategies, and enhanced claims forecasting, ultimately driving better financial outcomes and client satisfaction.
“This is especially true if we can tap into broader industry data for benchmarking purposes with our clients — something that we would not have been able to do in an automated format even just a few years ago.”
Weiss adds: “AI thrives on data, and data in captive insurance has the potential to provide rich insights. However, when people talk about AI models needing large amounts of data, they’re often referring to training neural networks from scratch — something that requires massive datasets far beyond what any individual captive insurer would have.
“In reality, the opportunity in captive insurance isn’t about building AI models from the ground up but rather leveraging existing, state-of-the-art AI models to analyse data and support better decision-making.”
He further explains that modern AI reasoning models can scrutinise claims behavior, aiding insurers in pinpointing opportunities for policy and process enhancements, detecting patterns, and making more informed decisions.
However, to produce truly valuable insights, AI requires more than just raw claims data — it needs context.
“Simply feeding claims history into an AI model will yield basic statistical correlations, which are far less insightful than the reasoning and judgment a human expert would apply. To achieve meaningful results, AI needs access to broader contextual data, including policy details and insured demographics and the knowledge of real-world events, to allow it to draw conclusions that are truly insightful,” Weiss notes.
?He argues that this challenge highlights a significant issue in the captive insurance industry: the lack of standardised data formats.
He notes that inconsistencies in the structuring of policies, insured information, and other contextual data hinder the effective application of AI across multiple firms or the industry as a whole.
Weiss concludes: "Standardising data formats would open the door for AI to provide far more powerful insights, benefiting not just individual captive insurers but the industry at large. Moving forward, the industry should prioritise creating common frameworks for data standardisation to fully unlock AI's potential."
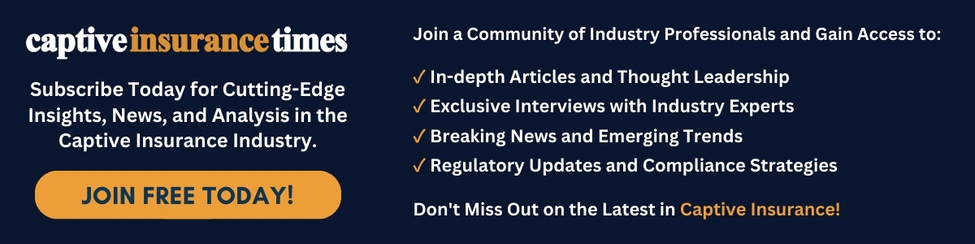